What’s The Best Attribution Model? The Only Guide To Attribution Tracking You’ll Ever Need
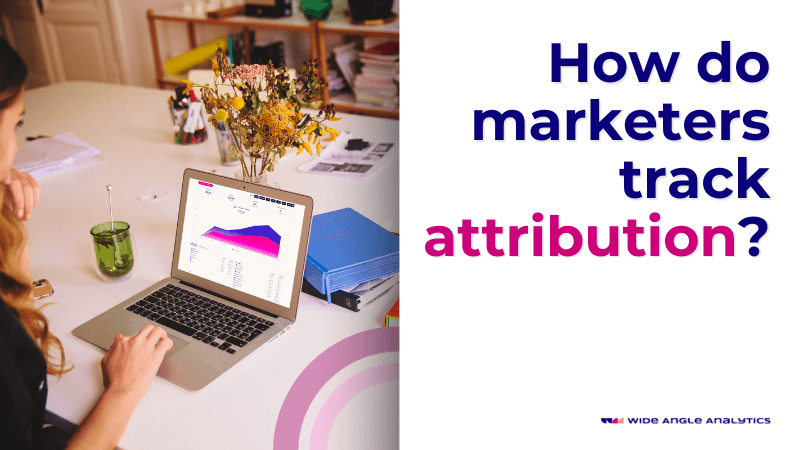
In the ever-evolving marketing landscape, choosing the most effective attribution tracking method can feel like charting unknown territory. This guide will teach you the essentials of attribution tracking trends and methods in 2023 to keep you up-to-date and help you select the right approach.
The modern marketing mix is complex. Nowadays, consumers interact with your brand via social media platforms, email campaigns, social media ads, Google display ads, your website and blog… And that’s without even mentioning traditional marketing methods such as TV, radio, billboards and magazine ads. How are you meant to unravel what’s having the biggest impact out of all these marketing channels?
Attribution tracking offers the answer to this complex question. In short, attribution tracking is the process of determining which clicks and impressions led to conversions.
There are numerous attribution methods to choose from, each putting a spotlight on different focal points of your marketing mix to try and help you decipher meaningful insights about what’s working and what’s not. Choosing the best attribution tracking approach for your strategy, preferences and goals is essential to glean genuinely valuable insights when analysing your campaigns. However, knowing which model to use is not straightforward.
Only 23% of marketers are confident they’re tracking the right KPIs. Online, queries and searches about marketing attribution have increased globally:
Rising Search Query | Global YOY % Increase in Online Searches |
---|---|
What is the purpose of attribution? | Breakout |
Last touch vs multi-touch attribution | Breakout |
Multi-touch attribution software | 300% |
B2B marketing attribution | 200% |
Marketing attribution tools | 90% |
Marketing channel attribution | 80% |
Attribution models marketing | 70% |
Attribution in marketing | 50% |
Attribution tools | 50% |
Multi-touch attribution model | 40% |
What is marketing attribution? | 40% |
To make things even more confusing, the introduction of cutting-edge AI technology, the transition to Google Analytics 4 (GA4) and other trends are reshaping the attribution landscape.
This guide will help demystify tracking attribution in 2023, including:
- Tracking attribution evolution and trends: how do marketers track attribution in 2023?
- A guide to attribution models: definitions, advantages, disadvantages, when best to use them and real-life examples.
- An attribution tracking model comparison table.
- Next steps for marketers looking to implement more accurate tracking attribution methods.
Attribution tracking trends: How do marketers track attribution in 2023?
Over the past year alone, online interest in attribution tracking has increased by 35%. New developments in AI alongside the rollout of Google Analytics 4 (GA4) have caused tracking attribution to be an even hotter topic than ever before. Keeping abreast of these tracking attribution trends is essential to stay ahead in the marketing game.
Here, we’ll explore the current trends having a significant impact on tracking attribution methods and what these mean for your own strategy.
The privacy revolution
In the not-so-distant past, third-party cookies played a pivotal role in the world of attribution tracking. These bits of code were the linchpin for identifying individuals across the vast expanse of the Internet, creating detailed profiles brimming with insights into their interests and behaviours. Creepy, right?
Thankfully, since then significant shifts have occurred in the way we approach online privacy. Nowadays, web browsers have measures to reduce the exposure of users' online browsing experience, fortifying privacy settings to thwart attempts at recognising users across different sites. This includes perhaps the most important action: blocking third-party cookies.
Today, users find themselves in a new digital dynamic, where decisions regarding third-party cookies are no longer theirs to make. Browsers have taken the reins, blocking cookies by default and ushering in an era of enhanced online privacy.
In this context, multi-touch attribution models find themselves at a crossroads. Enhanced online privacy is ultimately a good thing, but it does raise questions about how to reliably track and understand customer data while (quite rightly) respecting their browsing privacy.
Machine attribution models, such as algorithmic attribution, aim to solve this problem, which we’ll go into later in this article.
The rise of AI in attribution tracking
It seems there isn’t an area of marketing that goes untouched by AI’s impact. Tracking attribution included.
AI wields data-processing power that goes far beyond the capabilities of any human. It can sift through a vast sea of information that would otherwise be impenetrable, unveiling accurate and valuable insights and connections. In doing so, AI transcends the limitations of human analysis, unravelling patterns and relationships that were previously elusive.
AI can do this across extensive datasets — and from diverse sources. These intelligent models can even evolve and learn over time, honing their understanding of the various factors that impact events and conversions.
Perhaps the most remarkable aspect of AI's impact on marketing attribution is its ability to understand and accommodate external influences. From seasonality to competitor activity and macroeconomic trends, AI can dissect these elements to analyse and predict their sway over consumer behaviour.
Overall, AI presents a new era of precision, adaptability and foresight in marketing attribution and provides a more nuanced understanding of the customer journey
GA4 marks a paradigm shift in attribution tracking
In 2023, the transition to GA4 became official, as Google’s previous system, Universal Analytics (UA), was finally fazed out.
This was met with mixed feedback from marketers. On the one hand, the change was a necessary shift to a more sophisticated attribution model offering that could provide accurate insights in a privacy-first online world. But with this comes greater complexity in understanding the new attribution tracking methods.
For marketers and analysts, the shift to GA4 represents an opportunity to adapt to the changing digital landscape and lead the change in leveraging cutting-edge technology for more effective attribution tracking. But in order to do this, investment in time, training and consulting may be required to accurately utilise GA4 to its full potential.
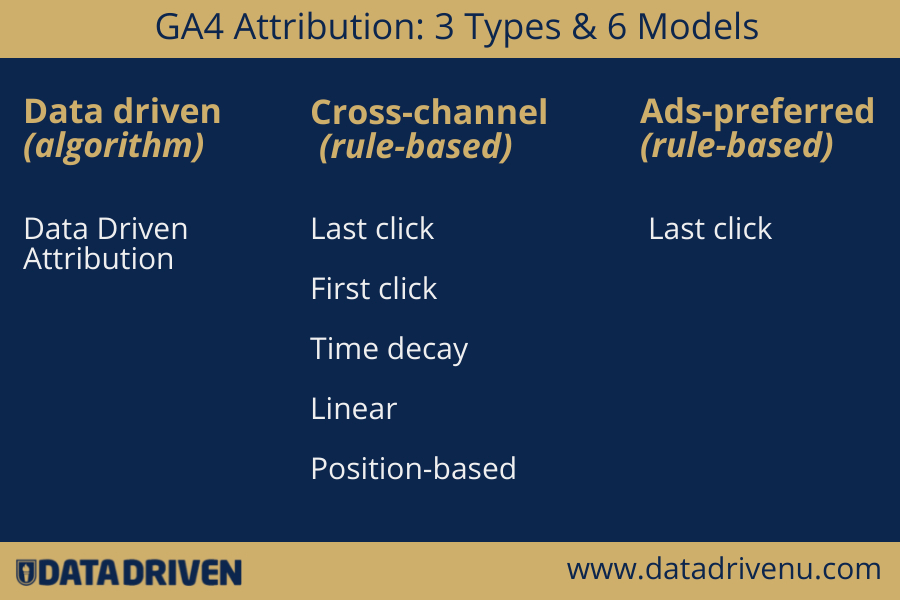
Navigating multi-channel marketing is increasingly complex
In today's dynamic marketing landscape, multi-channel marketing is the norm. The reality is that customers interact with a multitude of touchpoints before making a purchase decision.
Email marketing, PPC, organic social, website visits, paid social and more all contribute to the intricate tapestry of the customer journey. Understanding and assigning credit across these touchpoints is essential — and not straightforward.
Each individual embarks on a unique path to conversion, and quantifying the value of each touchpoint is necessary to understand where to invest more resources and which activities may require reevaluation.
This is where marketing mix modelling (MMM) steps into the spotlight, helping to decipher the nuanced interactions that lead to conversions. More about that later in this article.
The essential guide to attribution models in 2023
Now that you have an overview of the developments and trends making a splash in the tracking attribution space, it’s time to unearth the specific models marketers use to understand consumer interactions with a brand.
We’ll break down each attribution tracking model by its definition, advantages, disadvantages and when best to use it. Plus, we’ve included real-world examples of each one in action to show you just how valuable implementing the right attribution model can be.
Let’s get started!
First-touch attribution
What is it?
First-touch attribution credits the initial touchpoint that directed a customer towards a conversion. For example, if a customer clicks on an ad, explores your blog, subscribes to your newsletter and finally makes a purchase, under first-touch attribution the entire accountability will go to that initial interaction with the ad.
Advantages
- First-touch attribution is easy to understand and implement.
- First-touch attribution offers a clear window into the channels that are best at generating awareness and traffic.
Disadvantages
- First-touch attribution overlooks the influence of subsequent touchpoints in the customer journey, potentially missing valuable later contributors to a conversion.
- For long-term purchases, first-touch attribution may not capture the complexity and duration of the customer's path to conversion.
When best to use it
First-click attribution is most advantageous when you're trying to gauge which channels have the most significant impact in building brand awareness.
Example
In their first-touch report, HubSpot found their social channels (represented below by the teal bars) were responsible for nearly 3,400 leads and five customers during a single month. Paid advertising efforts (depicted in red) paled in comparison, highlighting the distinctive role of social media in introducing new leads to the company.
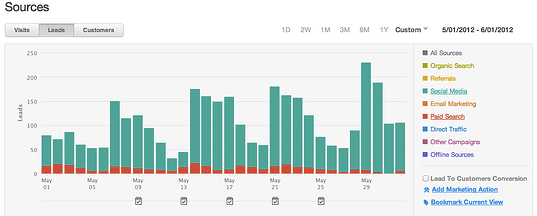
Last-touch attribution
What is it?
Last-touch attribution assigns the entirety of a conversion’s credit to the very last touchpoint that led the customer there.
Using the previous example again, if a customer engages with an ad, explores your blog, subscribes to your newsletter and finally makes a purchase, last-touch attribution credits the entire sale to that final interaction with the newsletter.
Advantages
- Last-touch attribution is simple and easy to implement.
- Last-touch attribution provides a lens into the channels that have the most influence in driving final conversions and sales.
Disadvantages
- Last-touch attribution overlooks the impact of earlier touchpoints that nurtured and persuaded the customer along their path.
When best to use it
Last-click attribution is best when you seek insights into the channels and touchpoints that wield the most influence in the final steps of the buyer's journey.
Example
Chris Walker, Founder of the marketing agency Refine Labs, talks about how last-touch attribution assigns more weight to conversions from organic traffic.
Using the last-touch attribution method reveals that 92% of his revenue stems from organic traffic, in contrast to survey results which suggest that 97% of his qualified pipeline emerges from social media, podcasts, word of mouth and community-driven channels.
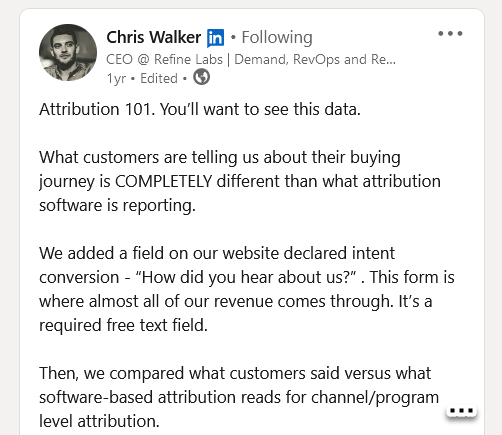
Multi-touch attribution
What is it?
Multi-touch attribution allocates partial credit to various touchpoints encountered along the customer’s path to conversion, employing different rules or models to weight the amount of recognition assigned to each channel.
Going back to our example customer journey where the customer engages with an ad, explores your blog, subscribes to your newsletter and eventually makes a purchase, multi-touch attribution would distribute credit among these touchpoints according to a predefined formula or weight.
Advantages
Multi-touch attribution paints a comprehensive picture of the customer journey and the unique impact of each touchpoint.
Disadvantages
- Multi-touch attribution can be more intricate and challenging to implement and analyse.
- Multi-touch attribution requires a wealth of data and assumptions, potentially introducing biases or errors into the mix.
When best to use it
Multi-touch attribution is best when you seek to dissect the influence of each stage in the customer journey.
Example
In a case study from LeadsRx, an enterprise harnessed multi-touch attribution to reduce marketing spend by over 44% without compromising their year-on-year revenue.
Algorithmic attribution
What is it?
Algorithmic attribution uses the power of machine learning (ML), whereby machines are developed with the capability to discover algorithms by themselves, without human interjection. Unlike simplistic formulas, this method dives deep into vast datasets from diverse sources to accurately allocate credit for each touchpoint leading to a conversion.
Advantages
- This accurate approach is the best way for marketers to fully optimise spending, guiding decisions on budget allocation and pinpointing areas for reduction.
- Algorithmic attribution enhances personalisation in marketing strategies, messaging and product offerings.
Disadvantages
- Implementing algorithmic attribution can be a formidable challenge. Organisations must effectively collect a wealth of comprehensive data from a multitude of sources for algorithmic attribution to work.
- Processing and analysing the huge volume of data required for algorithmic attribution can strain legacy systems.
When best to use it
Algorithmic attribution is best when you seek the most comprehensive and accurate depiction of the customer journey and you have copious amounts of data or long and intricate customer journeys.
Example
Online fashion store Thread implemented an ML attribution model to combine cross-channel data into one streamlined overview of their entire marketing mix. The resultant attribution provided fair and detailed weighting of all Thread’s activities, allowing the marketing team to accurately analyse which channels contributed the most to sales and revenue.
Armed with this new knowledge, Thread maximised upon untapped top-of-the-funnel (TOFU) opportunities and increased new customer acquisition by 16% in just 6 months.
Marketing mix modelling
What is it?
Marketing mix modelling (MMM) uses statistical analysis to allocate the influence of each marketing method on consumer behaviour, sales and ROI. Essentially, MMM establishes the relationship between a variable (like a marketing or sales activity) and an outcome (such as sales or profit). This illuminates the web of cause and effect within marketing strategies.
Advantages
- MMM provides a holistic understanding of your target audience and their journey, shedding light on the role played by a full range of touchpoints, like ads, radio and TV.
- MMM guides you in optimising your marketing spend to yield maximum results and avoid diminishing returns.
Disadvantages
- MMM is designed to determine how much to allocate to each channel, but not the specific strategies or vendors to employ.
- Implementing MMM demands a substantial commitment of time and resources.
- The relationship between investment and conversions isn't always linear — i.e. a 10% investment doesn’t always lead to a 10% increase in conversions. This can pose a challenge when deducing MMM insights.
When best to use it
MMM excels in campaigns where the goal is to understand the driving forces behind sales and profits across various contributors, allowing for better budget optimisation.
Example
While this isn’t a specific case study, Google's article describing the application of MMM in the travel industry shows its potential and efficacy. Google highlights how MMM can provide the travel sector with data-backed insights relating to organic and paid search, leading to more informed and impactful marketing strategies.
Data-driven attribution
What is it?
Data-driven attribution focuses on user interactions with ads, discerning which keywords, ads and campaigns exert the most significant impact on your business objectives.
Advantages
- Data-driven attribution unveils the role played by keywords, ads, ad groups and campaigns, enabling you to fine-tune bidding strategies.
Disadvantages
- Data-driven attribution doesn't provide a granular breakdown of how each channel contributes to a sale.
When best to use it
Data-driven attribution excels in assessing your digital advertising campaigns, guiding you towards the best bidding strategy.
Example
Target's experience with data-driven attribution shows its potential. By shifting away from last-click attribution, Target gained invaluable insights into the real value of keywords used by customers in their research phase. Switching to data-driven attribution resulted in a 66% surge in conversions and a 1.6x increase in return on ad spend (ROAS).
Attribution tracking model comparison table
As we’ve discovered, the attribution tracking model you choose can significantly impact your understanding of customer behaviour and, ultimately, the success of your campaigns. Here, we’ve created an easy-to-use comparison table for you to quickly compare attribution tracking methods, helping you decide which is best for your situation and needs.
Attribution Method | What is it? | Advantages | Disadvantages | When best to use it |
---|---|---|---|---|
First-Touch Attribution | Attributes all credit for a conversion to the first touchpoint that initially engaged the customer. | Easy to implement and understand. Identifies effective channels for generating awareness and traffic. |
Ignores the influence of other touchpoints. Doesn't account for the complexity of the customer journey. Less suitable for high-value or long-term purchases. |
When focusing on measuring channels that build brand awareness and first interactions with the business. |
Last-Touch Attribution | Attributes all credit for a conversion to the last touchpoint that occurred before the customer converted. | Easy to implement and understand. Identifies effective channels for driving conversions and sales. |
Ignores the influence of other touchpoints. | Doesn't account for post-conversion interactions like retention and brand advocacy. |
Multi-Touch Attribution | Assigns partial credit to multiple touchpoints along the customer journey, based on different rules or models. | Provides a comprehensive view of the customer journey. Helps optimise marketing mix and budget allocation. |
More complex and challenging to implement and analyse. Requires more data and assumptions, which can introduce bias or error. |
When seeking to understand the influence of each touchpoint and to optimise marketing strategies across channels. |
Algorithmic Attribution | Utilises machine learning to analyse large data sets and determine credit for conversions. | Provides the most accurate view of top-converting channels for you to optimise marketing spend. Enables improved personalisation. |
Requires access to large and diverse data sets. May be challenging for organisations lacking comprehensive data sources. Demands significant computational power and storage capabilities. |
When aiming to get a precise view of the customer journey, particularly when you have abundant data or complex customer paths. |
Marketing Mix Modelling | A statistical analysis technique to evaluate the impact of each marketing input on consumer behavior and ROI. | Evaluate the impact and effectiveness of various marketing efforts to optimise marketing spend. Helps understand the role of all touchpoints in the buyer’s journey. |
Doesn’t provide specifics on how or with which vendor to allocate spend. Requires significant time and cost investment. Non-linear effects may impact accuracy. |
When looking to understand the impact of different marketing activities and drivers on sales and profitability. |
Data-Driven Attribution | Analyses customer engagement with various ads to give credit for conversions. | Offers a data-based approach for evaluating the impact of ads and optimise bidding. | Does not show how each channel gets credit for a sale. | When measuring advertising campaign success and determining which channels have the highest impact across the web. |
Choosing your attribution model
If there’s one thing to take away from this article, it’s that there’s no single definitive attribution model for all businesses. What's best for your business may not be the same for another. Your unique circumstances, audience behaviour and campaign goals should guide your decision — and it’s crucial to get it right.
By selecting the right attribution model, you unlock valuable insights into your marketing strategy: what channels have the most impact on awareness, conversions, sales and profit, and where to allocate time, money and resources. So, whether you're a CMO steering the ship or a Business Analyst deciphering the data, make sure you choose your attribution path wisely.
Luckily, armed with the knowledge gained from this comprehensive guide, you’re better equipped to make informed decisions that will deliver real results for your company.
Understand your audience better with Wide Angle Analytics
Unravel complex customer journeys and gain the knowledge you need to make informed decisions. Wide Angle Analytics helps businesses thrive in a data-conscious world, providing invaluable analytics insights while meticulously adhering to international user privacy laws.
Leveraging state-of-the-art technology and a deep understanding of privacy regulations, Wide Angle Analytics’ website analytics software ensures you can track and attribute user interactions effectively — while never compromising personal data.
Click here to learn more about accurate, privacy-first web analytics with Wide Angle Analytics.
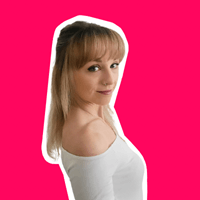
Lauren Meredith is a seasoned content marketing strategist and writer helping online businesses connect with their audience and maximise organic success. Her SEO content secures #1 positions on Google, features in publications such as The Independent, Yahoo and academic domains, and has won an award at the Digital Growth Awards.